In today’s rapidly digitizing world, the importance of data security has become paramount. With the increasing amount of sensitive information being shared and stored online, securing information from cyber attacks, information breaches, and theft has become a top priority for companies of all sizes. Data loss prevention (DLP) is a critical part of the Netskope Intelligent Security Service Edge (SSE) security platform, providing best-in-class data security to our customers.
Images often contain a wealth of valuable and sensitive data. Financial documents, personal identification, and confidential business communications frequently include images that require the utmost security. At Netskope, we have developed state-of-the-art deep learning-based computer vision classifiers that can analyze images and identify sensitive information in a wide variety of categories such as passports, drivers licenses, credit cards, and screenshots. We have been awarded four U.S. patents for our innovative approach to data security. In this blog post, we highlight recent improvements to our image classifiers that resulted in higher accuracy and better customer experience.
CNN Architecture Update
At the heart of our image classification models lie convolutional neural networks (CNNs). These powerful deep learning algorithms are specifically designed for image recognition and classification tasks. By employing a technique known as transfer learning, we take advantage of pre-existing CNNs that have been trained on large-scale datasets and fine-tune them using a smaller dataset of labeled images that contain sensitive information. As a result, our classifiers are able to quickly identify the unique patterns associated with the sensitive information, with high accuracy and reduced training time.
There are several practical concerns in selecting the pre-trained CNN models. Given that our classifiers are used to scan millions of customer files daily by our SSE platform, it is crucial to keep false positives as low as possible to avoid overwhelming customers with spurious alerts. Simultaneously, since true positives indicate a serious data leak, maintaining a high true positive rate is equally important. An additional challenge lies in creating classifiers complex enough to meet our accuracy goals yet compact enough to fulfill our stringent latency requirements, since they run in real time on the SSE platform. As such, we only considered pre-trained CNN model architectures with fewer than 10 Million parameters.
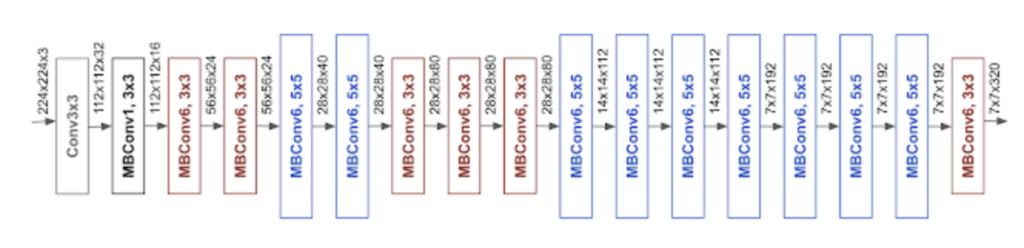
In our latest model update, we transitioned to EfficientNet pre-trained CNN architecture (modeled in the figure above). This lead to an 80% increase in the number of model parameters. Using a larger pre-trained model incurred a modest increase in latency but yielded a significant boost in real-world accuracy.
Training on real cloud data
In order to minimize false positives, it is important for our image classifiers to be exposed to a wide variety of realistic negative samples. To achieve this, we have sourced tens of thousands of actual cloud images from our own corporate data. This approach enables us to collect a substantial number of genuine training images, while simultaneously maintaining our commitment to customer privacy. These images were labeled by hand, with the majority of them being either negative examples or screenshots typical of real-world cloud data.
In addition to these random negative examples, we have also incorporated several thousand carefully curated adversarial samples, further bolstering our classifiers’ resilience against false positives. One interesting type of adversarial sample was labels for electronics. Due to their bold fonts and high contrast coloring, they can be mistaken for sensitive documents. By training our classifiers on these adversarial examples, we can effectively prevent such misclassifications in the production environment.
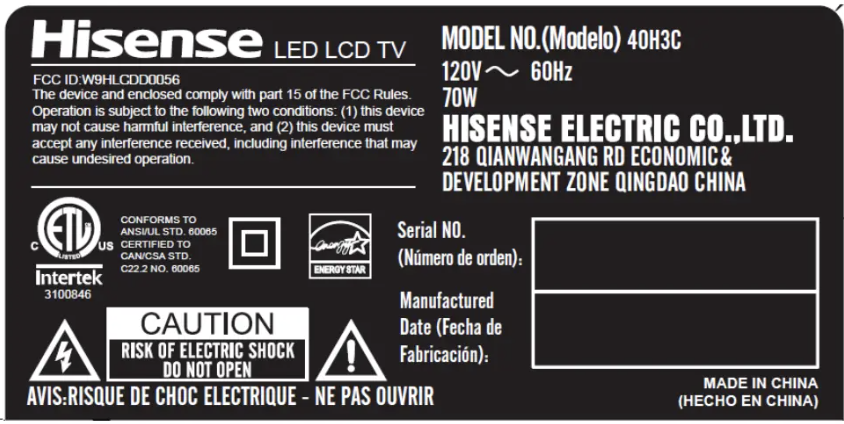
Custom data augmentations
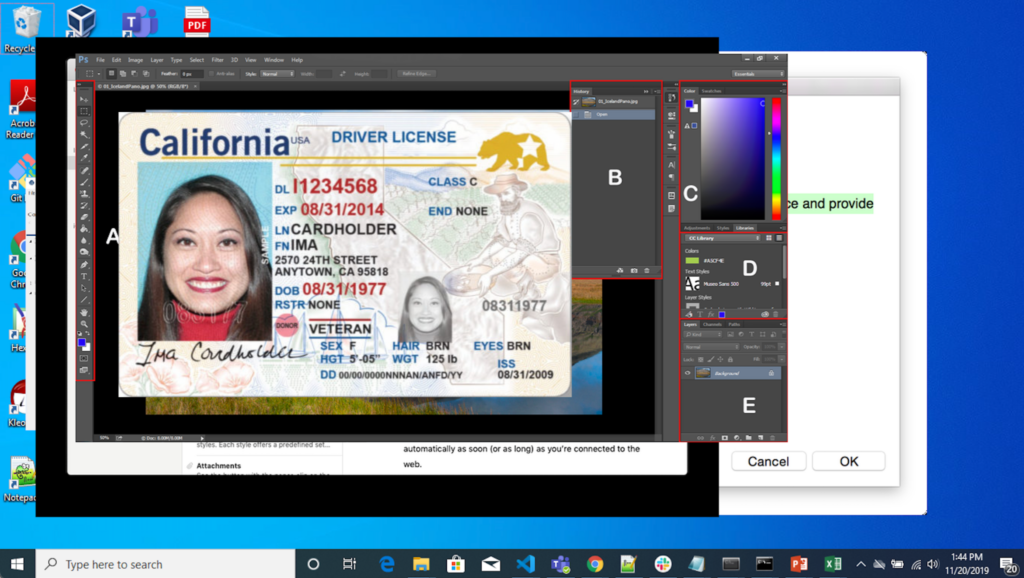
In addition to sourcing real cloud data, we employ a comprehensive suite of data augmentation techniques specifically designed for computer vision applications, such as rotation and cropping. What sets our approach apart is the customization of these augmentations to ensure maximum fidelity with the image data encountered in real cloud environments. One example is our custom augmentation that seamlessly integrates documents onto realistic backgrounds, such as a driver’s license pasted on a screenshot. This enables our classifiers to train on documents in a diverse range of settings, significantly boosting its versatility and performance on real-world data.
Summary
In our pursuit to develop cutting-edge AI security solutions, we continuously strive to refine our methodologies and data sources to build powerful, adaptive data security models capable of safeguarding the ever-evolving digital landscape.
To learn more about how Netskope helps customers protect their sensitive data everywhere across their entire enterprise, please visit Netskope Data Loss Prevention. And to keep up with with what our AI Labs team is writing about, please visit our AI Labs blog page here.